A Roadmap to AI Adoption
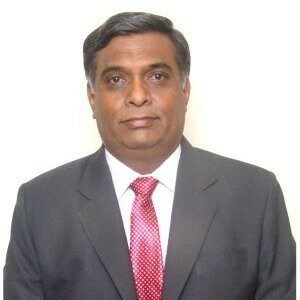
Sudhakar Nagasampagi
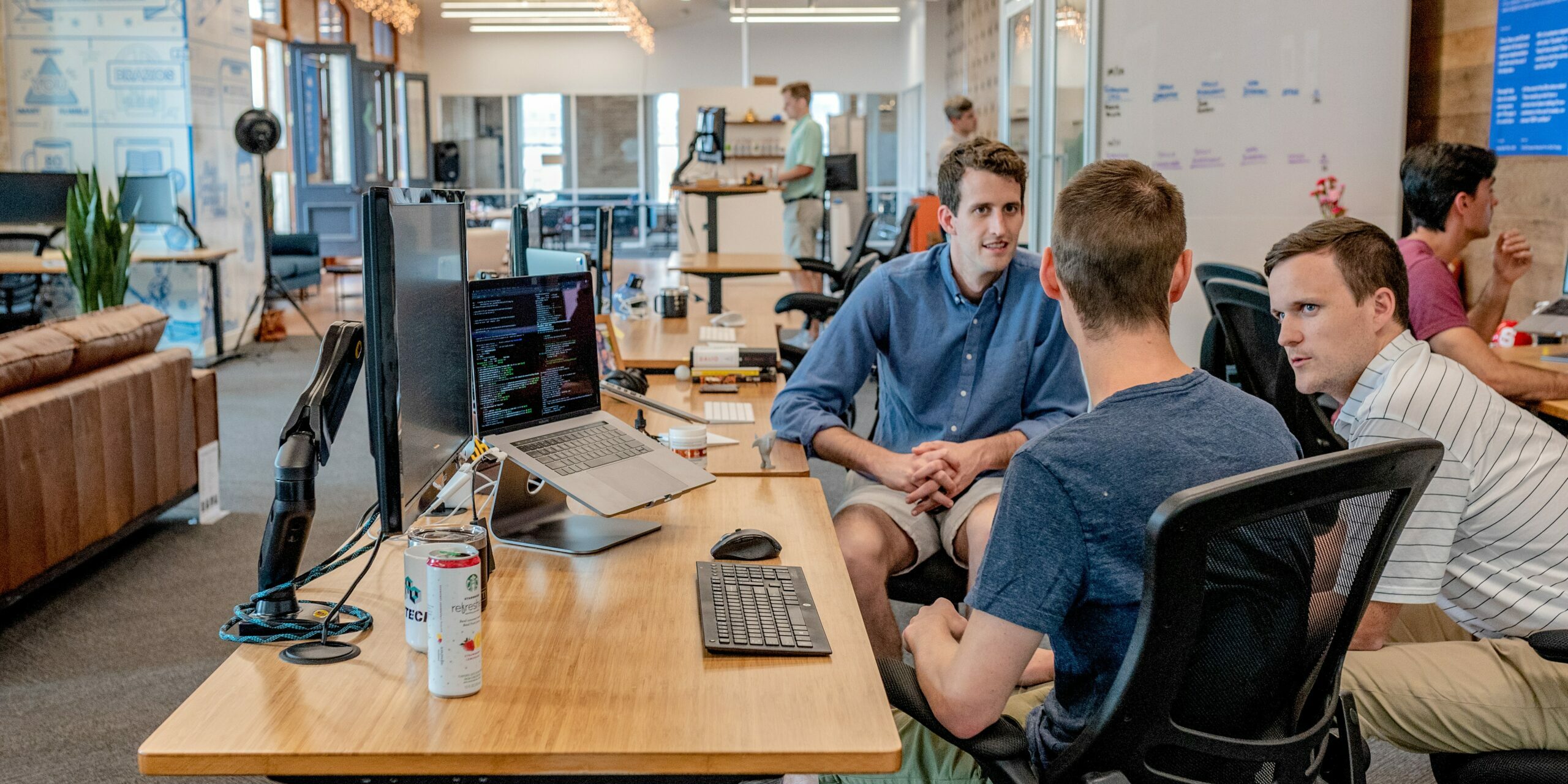
A Roadmap to AI Adoption
AI adoption is not achieved by using a simple or straightforward formula or process. Surveys today indicate that only 10% of the organizations worldwide engage in the core use of AI technologies while the remaining run only ad hoc pilots and simple AI-related business applications.
In this article, we will go over real-world examples of AI in organizations, we’ll also explore the risks and challenges to which they can be confronted, and finally uncover the inherent work on the cultural change and the prerequisite skills that the individuals in organizations should get ready to assimilate. Let’s dive into it.
Table of Contents
Real-world applications of AI in organizations, what do we learn?
Let’s have a look at a couple of well-reputed, global visionaries who, despite having spent billions of dollars, failed in their initial efforts. Why did they fail? In this part, we examine the critical success factors required for AI adoption.
Here are some examples of several epics and not so epic failures of prominent organizations, which, with much fanfare, started their AI journey just to find their dreams shattered and goals unattainable.
- IBM partnered with doctors and cancer centers and used AI, with an investment of $62 million, for their product “Watson” to diagnose and treat cancer patients. The product, however, provided major erroneous and dangerous treatment recommendations due to which the project had to be abandoned. IBM did not realize that the hypothetical tests that were carried out would not be an equivalent treatment to real-life situations.
- Microsoft made big headlines when unveiling their chatbot, “Tay” which was supposedly meant to chat with people and become smarter the more it engaged and conversed. Unfortunately, people tweeted crude, vulgar and racist remarks at the bot. In a matter of hours, Tay itself started using foul and offensive language and Microsoft had to call off this venture. Microsoft was not prepared for this kind of failure – actually, it was more of a catastrophe.
- Amazon scrapped their AI-developed hiring process project when they realized that it was biased against women.
- Facebook shut down their two chatbots “Bob” and “Alice” when it found that they developed their own chat language which people claimed was scary and total jargon.
- The famed Apple released its iPhone X with much aplomb and boasted of Facial Recognition, one of the key capabilities. However, this very feature failed when it got fooled by a human wearing mask and was unable to unlock the phone. Another major flaw detected was its inability to differentiate between identical twins.
- It was observed that Google Home Minis had been secretly turning on and recording thousands of minutes of audio of their owners, and sending the tapes to Google. Once confronted, Google quickly announced a patch to prevent the issue.
- An ethical debate sparked and still continues as to who was responsible for the death of a pedestrian in the US killed by an unmanned Uber’s self-driving car.
After this list of (near) total disasters, something easily comes to mind, what makes companies such as Google, Facebook, Amazon, Netflix, and Spotify so popular?
These companies have been the front-runners to develop a vision and a strong operating model for AI adoption. The execution of the operating model and the realization of the vision has not been an easy journey; rather, one wrought with many known and unknown obstacles. Repeated experimentation through analysis, testing, and re-testing over long hours, days and months has finally led to varying degrees of success in AI adoption and implementation.
So, the question boils down to the following inference: If an organization has a vision and an operating model, can success be guaranteed? Any technology that you plan to adopt cannot be achieved by assembling an ad-hoc business case, a hurried development process, and surface-level testing.
The need of the hour is to first really understand whether AI will be a difference-maker to the bottom line of the organization’s business. Next is to evaluate the current market conditions, and how other businesses and competitors are making use of AI – especially the lessons learned through their mistakes/failures during their AI adoption journey. Then is to identify the benefits and risks associated with this adoption. Further, create a long-term strategy (vision), a matching operating model, and initially, start experimenting with a small-scale model. This and further experimentations should lead to the next levels of adoption.
Recognize the risks and likely disruptions and have adequate risk management and exit strategy
Any strategy document on adopting AI necessarily needs to be conscious of the probable factors of the AI ecosystem that may undermine ethical conduct, impinge on one’s privacy and undermine the security protocol. Appropriate steps to manage and mitigate these risks need to be an integral part of any such strategy. Proper risk identification, assessment, and treatment can speed up the pace of adoption. Generally speaking, it is always a good practice to adopt a well-known risk management framework.
We can start with one of the major issues in AI: Ethics – fairness or tackling different types of biases. Data that is inherently collected and being collected may have biases, which can get reinforced over time. A possible way to address this issue is to identify the in-built biases, assess their impact, and in turn discover ways to reduce the bias.
Another important challenge is to ensure data privacy. Privacy has been termed as a fundamental right. People often unknowingly give consent to sharing their data which they would not have ordinarily done had they known the purpose their data were being put to. There is an urgent need to spread awareness among individuals about the importance of consent, ethics, and privacy while dealing with technology. Concerns arise when companies harvest significant amounts of consumer data and use it inappropriately to gain insights about consumers. The key here is that the consumer may not have access to these insights or the ability to derive value from them. Companies should aspire or be directed to become more transparent on how they use consumer information, the value they provide in return, the period of data retention, and the manner in which such data is disposed of or destroyed. Other ways to address privacy issues are to establish a data protection framework with legal support, benchmark data protection and privacy laws with international standards such as GDPR, spread awareness amongst consumers to share only mandatorily required personal data with authorized entities such as Tax Returns, Passport / Visa offices, Banks, and so on.
The security aspect in AI is yet another critical challenge that should be addressed. In healthcare, an incorrect diagnosis prediction and corresponding treatment can lead to severe complications or even cause loss of life. Similar can also be the result of a wrong maneuver in driverless vehicles. Every failure needs to be thoroughly investigated, and a suitable course of action laid out in order to prevent future incidents.
A well-tested incident response plan will help to recover timely from any untoward disruptions or disasters. Key stakeholders should be identified and alerted when any incident occurs. If there has been a breach, the cause should be ascertained and the regulatory bodies, as well as the public, should be made aware of the same.
Finally, an exit plan can assist in alternate approaches should the project run into troubled waters. Discretion should be exercised in certain cases where organizations should accept the fact that there is no justification in the argument that since a lot has already been invested, the project should continue to strive in the hope of seeing light.
Adopting AI is a cultural journey leading to the change in the structure of the organization
By nature, organizations and humans are resistant to change. The stumbling block today is that organizations’ cultures are almost the exact opposite of what they need for the fast and wholehearted adoption of AI-powered business models. Research shows that the most critical challenges stand with people and the organization’s culture, the 2 first ranked to be “Employees prefer to stick to their tried and tested routines” and “Employees are afraid to lose their jobs if these technologies take over.”
It, unfortunately, shows that most organizations don’t have a commonly directed and understood vision, don’t encourage people to question decisions, don’t handle unpredictability and uncertainty well, and don’t cultivate environments of trust and ethical accountability — at least, not to the extent that they are required.
All the stakeholders in the organization play an important part in formulating the vision, necessary objectives, and strategic plans from the leadership to operating teams. Primarily, stakeholders must be first prepared by having a foundational understanding of AI. To ensure AI adoption, everyone in the organization should be educated, aligned on the mission.
Employees should be made aware of the implications of introducing AI in the organization. They must understand why AI is important to the business and how it will fit into a new, AI-oriented culture. They should be encouraged, from the beginning, to ask open questions and take part in discussions and development, allaying any fears that their jobs are at stake. Workplace relations should be based on trust and win-win thinking. If required, organizations may be restructured to accommodate the new roles and skills.
More importantly, companies should be ready to set up a learning strategy, setting up technology workshops, training sessions, and other educational courses for employees. By encouraging their workforce to develop skills associated with the technology advancement they are choosing to apply, they will build a stronger culture and mindset around these new technologies removing the fears of change and including the employees in the change process.
Explore the prerequisites required for working with AI
Working with AI requires both business and/or technical level knowledge and experience. Business associates including senior management focus on how best to garner the benefits of AI with respect to the organization’s vision and objectives. This and how to deal with risks and challenges are dealt with in more detail in the next sections.
From the aforementioned research, the 4th ranked critical challenge was the “ Lack of skilled employees for AI or advanced analytics related tasks”. It becomes essential to investigate which are these skills generally needed and for organizations to propose or if anything encourage their employees to individually take courses and certifications in AI.
In this section, we focus on the technical aspects required for developers and implementers of AI sets of technologies.
Primarily, the questions that crop up for technical professionals to work with AI are
- Do I need to know coding or programming? Is it sufficient if I have just understood the concepts of programming or do I need to have really done coding or programming in real-life applications? What design patterns or languages are commonly required when working with AI?
- Is it necessary to be well versed with Object-Oriented Programming (OOP) architectures and principles?
Programming is just one aspect that is important when dealing with AI applications. More importantly, certain areas that fall under the prerequisites are:
- Well-versed with concepts of Information Technology (Computing, Networking, Security, Privacy, and others)
- Well-versed with an object-oriented programming language
- Strong understanding of Algorithms and Data Structures
- Strong understanding of Big Data types, Data Analytics lifecycle, and Data Visualization
- Strong understanding of Relational and Non-relational databases, data lakes, and data queries
- Good understanding of Mathematics (Slopes, Graphs, Derivatives, and Calculus)
- Good understanding of Statistics (Basic Concepts, Measures of central tendency and dispersion, Distribution types, Probability)
- Familiarity with Cloud Computing (Concepts, characteristics, models, benefits and challenges)
- Familiarity with Agile principles and methodologies (Scrum / XP / TDD)
- Familiarity with DevOps practices
- Overview of emerging technologies such as IoT, RPA, and others.
AI requires a lot of experimentation, and many times, the early iterations do not work out as planned. In such cases, leaders must actively encourage sharing of experiences and opinions regarding the causes and likelihood of such failures. They should motivate the right people to come up with new or alternate ways that can address such issues and also continually reinforce the organization’s vision and goals.
It is a long road ahead for organizations transforming to become fully AI-driven, involving a long process of change in technology, people, and culture. Considering that AI-beginners will have stronger barriers to change, it is essential to explore the training possibilities in order to assemble the whole organization in a mindset of collaboration and learning.
All in all, it is important for organizations to cultivate the right culture for adopting AI because cultures, unlike technologies, are difficult to change rapidly. Further, business units must lead AI projects and be both accountable and responsible for the success.
Courses to help you get
results with Artificial Intelligence
Artificial Intelligence Foundation™
The CCC’s Artificial Intelligence (AI) Foundation™ course provides an overview and insight into the critical emerging technology of Artificial Intelligence to organizations and individuals around the world. This vendor-neutral, cross-industry foundation course: Builds upon the AI definition, its evolution, concepts, and applications. Provides an introduction to machine learning concepts. Explains the main business drivers and…
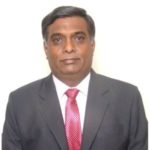
About the Author
Security Specialty Trainer; AWS Architect; CCC Master Trainer, Author of CTA, CTA+ & IoT; and Accredited DASA DevOps Trainer with 25+ years of IT experience.
Never miss an interesting article
Get our latest news, tutorials, guides, tips & deals delivered to your inbox.
Keep learning
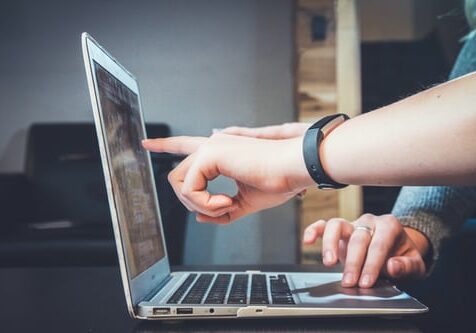